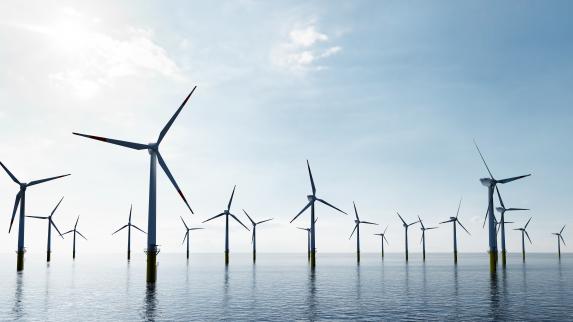
Adobestock.
The wind energy industry could soon count on a much-needed precise analysis to achieve an optimal balance for wind farm productivity and profitability, thanks to a team of researchers working with digitization, predictive and prescriptive analytics to bring down its operational costs.
Rutgers researchers led by Principal Investigator Ahmed Aziz Ezzat, assistant professor of Industrial & Systems Engineering at the School of Engineering, and Co-Principal Investigator Joseph F. Brodie, Offshore Wind Research Lead and director of Atmospheric Research at the Rutgers Center for Ocean Observing Leadership are teaming up with researchers from Wayne State University, Argonne National Laboratory, and Cognite. Their ultimate goal is to bring data and decision sciences closer towards meeting the ambitious 35%-by-2050 U.S. wind energy target.
Rutgers and Wayne received a $450,000 grant award from the National Science Foundation (NSF) for their academia-industry collaborative project that will run for three years. Wayne’s Principal Investigator is Murat Yildirim.
“We aim to formulate a decision-theoretic approach to jointly optimize generation and maintenance in wind farms, taking into account the uncertainties that could arise from weather variability, generation of electricity and profits, and the degradation of key turbine components,” said Ezzat. “For wind farm operators, wind is both the promise and the peril – it is the primary resource of electricity generation and hence, profits, but is also the main driver of structural degradation, and ultimately, maintenance expenses. We will re-envision a challenging generation-degradation trade-off in the wind industry through a data science and systems engineering lens.”
The US Office of Energy Efficiency and Renewable Energy plans to accelerate the transition to a net-zero greenhouse gas emissions ensuring clean energy while creating jobs. According to the Office, by 2050, wind power could fund more than 600,000 jobs in manufacturing, installation, maintenance, and other services. A 2020 report from the Department of Energy indicated a record growth in land-based and offshore wind energy.
While Ezzat acknowledges that wind energy still faces barriers related to its operations and maintenance (O&M) costs, he reaffirms that the industry is attractive and competitive relative to conventional fossil-fuel energy sources. And he says it needs better models and solutions to become more cost-efficient.
“We’ll search for timely solutions to enhance the operational efficiency in wind farms, thus facilitating wind energy’s cost-effective integration into the U.S. electricity grid,” added Ezzat. “The industry has been primarily driven by the economic value of operations and maintenance decisions. Our proposed ideas align well with the farm operators’ mindset and, hence, have the potential to reshape the prevalent single-faceted O&M strategies which have largely overlooked the dependencies between the generation and degradation processes in wind turbine assets.”
The main ingredient of the data science solutions, according to Ezzat, is the rich information collected by sensors in modern-day wind farms including historical SCADA records, weather variables, structural health parameters, and other data. The research team will create digital representations of how the turbines have been working, are currently working and how they could be more productive to reliably generate electricity under various weather conditions while ensuring optimal maintenance at the best possible time.
As part of the project, the researchers will use data obtained through wind farms in other parts of the world through an agreement with a major wind energy developer headquartered in Europe.
The collaboration with Cognite, a leader in industrial software innovation for the full-scale digital transformation of asset-heavy industries, will accelerate the translation of the proposed data science solutions into tangible industrial impact.
“Our core Industrial DataOps platform, Cognite Data Fusion™, liberates and contextualizes data for quick collaboration on the development, operationalization, and scale of industrial AI solutions and applications. This project with Rutgers is an exciting opportunity to leverage emerging technologies like hybrid AI, machine learning, and 3D modeling to develop holistic, sustainable, and scalable solutions in the wind market,” said Mina Mousa, Co-PI and Senior Data Scientist at Cognite. “We’re tackling predictive maintenance and power production optimization simultaneously to drive overall cost of operation to the absolute minimum, which can have a big impact on the growth of wind energy moving forward.”
“Data science has the potential to help us to better generate power,” added Ezzat. “We can reliably and cost-effectively power up and generate clean energy if equipped with the right data science tools.”
This article first appeared on Rutgers Research news.